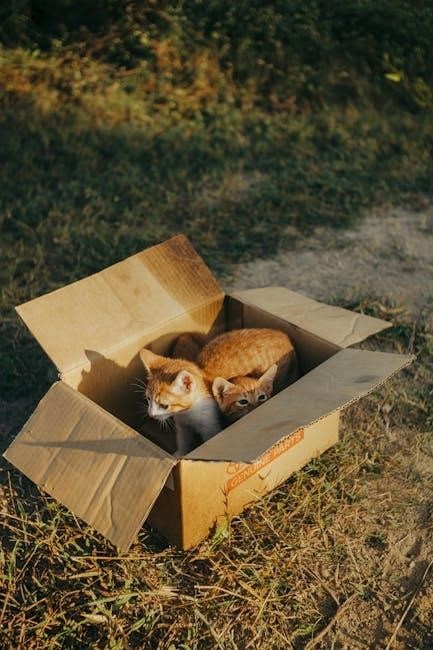
-
By:
- jayson
- No comment
box and whisker plot explained pdf
A box-and-whisker plot is a visual tool that displays the distribution of data, showing the median, quartiles, whiskers, and outliers. It helps compare datasets and understand variability.
Overview of Box and Whisker Plots
A box-and-whisker plot is a graphical method for displaying the distribution of a dataset. It shows the minimum, maximum, median, and quartiles, providing insights into data spread and central tendency. Whiskers represent the range, while outliers are highlighted beyond the whiskers. This tool is widely used for comparing datasets, identifying skewness, and detecting anomalies, making it a clear and effective way to visualize data distribution and variability.
Definition and Explanation
A box-and-whisker plot is a graphical tool in statistics that displays the median, quartiles, whiskers, and outliers of a dataset, aiding in data distribution analysis.
Understanding the Components
A box-and-whisker plot consists of a box, whiskers, and outliers. The box represents the interquartile range (IQR), containing the median (Q2) and quartiles (Q1 and Q3). Whiskers extend to show data range, with outliers beyond 1.5IQR marked separately. This structure visually highlights data spread, central tendency, and anomalies, aiding in understanding data distribution and variability effectively.
Statistical Measures in Box Plots
Box plots display key statistical measures: median (Q2), lower (Q1) and upper quartiles (Q3), interquartile range (IQR), and range. The median divides data into halves, while quartiles split it into quarters. IQR measures middle 50% spread. Whiskers show data range, with outliers beyond 1.5IQR. These measures help assess central tendency, spread, and asymmetry, providing insights into data distribution and variability effectively.
History and Development
Box-and-whisker plots were introduced by John Tukey in the 1970s as part of exploratory data analysis. Initially called “skep plots,” they evolved to become a standard visualization tool;
Origins of the Box and Whisker Plot
Origins of the Box and Whisker Plot
The box-and-whisker plot was introduced by John Tukey in the 1970s as part of exploratory data analysis. Initially called “skep plots,” they were designed to visualize data distributions. Tukey’s innovation laid the groundwork for modern statistical visualization, emphasizing understanding data spread and central tendency. Over time, the name evolved to “box-and-whisker plots,” becoming a standard tool in statistics and education for comparing datasets and identifying outliers.
Evolution in Usage and Interpretation
Box-and-whisker plots gained popularity in the 1980s as a tool for exploratory data analysis. Their interpretation evolved to emphasize understanding data spread, central tendency, and outliers. Advances in statistical software made them easier to create, leading to widespread use in education, research, and industry. Today, they are a standard method for comparing datasets and visualizing distributions, aiding in inferential reasoning and decision-making across various fields.
Key Components of a Box and Whisker Plot
A box-and-whisker plot consists of a box, whiskers, median, quartiles, and outliers. The box shows the interquartile range, whiskers extend to data extremes, and outliers highlight unusual values.
The Box: Median and Quartiles
The box represents the interquartile range (IQR), containing the middle 50% of data. The horizontal line inside the box is the median, dividing data into upper and lower halves. The edges of the box mark the first (Q1) and third (Q3) quartiles, indicating data distribution and central tendency.
The Whiskers: Range and Outliers
The whiskers extend from the box to show the range of data, excluding outliers. They typically reach 1.5 times the IQR from Q1 and Q3. Points beyond the whiskers are outliers, indicating unusual data points. This feature helps identify data extremes and potential anomalies, enhancing understanding of the dataset’s spread and variability.
When to Use Box and Whisker Plots
Box and whisker plots are ideal for comparing distributions, identifying outliers, and understanding data spread. They are particularly useful for visualizing multiple datasets and assessing variability.
Comparative Analysis
Box and whisker plots are highly effective for comparative analysis, allowing side-by-side visualization of multiple datasets. They enable easy identification of differences in medians, quartiles, and ranges. This makes them ideal for comparing groups, such as test scores across classes or product performance between brands. Double box-and-whisker plots further enhance this capability, providing a clear visual contrast to highlight variations in data distribution and central tendency.
Detecting Outliers and Skewness
Box and whisker plots are valuable for identifying outliers, which appear as points beyond the whiskers. They also reveal skewness through the position of the median relative to the quartiles. If the median is closer to one quartile, the data is skewed. Outliers and skewness are visually apparent, making box plots a robust tool for assessing data distribution and anomalies without complex calculations.
How to Create a Box and Whisker Plot
Organize data, calculate quartiles, median, and extremes. Plot the whiskers, box, and outliers. Use manual steps or software for clarity and precision in data visualization.
Manual Creation Steps
To manually create a box-and-whisker plot, start by ordering the data set. Calculate the first quartile (Q1), median (Q2), and third quartile (Q3). Determine the interquartile range (IQR) by subtracting Q1 from Q3. Identify the lower and upper whiskers, typically 1.5*IQR from the edges of the box. Plot the data on a number line, draw the box between Q1 and Q3, add whiskers, and mark outliers. Label the axes and ensure clarity in representation.
Using Statistical Software
Statistical software simplifies box-and-whisker plot creation. Tools like Excel, R, Python, and SPSS allow users to input data and generate plots automatically. In R, the boxplot
function creates plots, while Python’s matplotlib
and seaborn
libraries offer customizable options. These tools calculate quartiles, medians, and whiskers, identify outliers, and produce visually appealing graphs. They save time and reduce errors, enabling quick data visualization and analysis for informed decision-making.
Interpreting a Box and Whisker Plot
Interpreting a box-and-whisker plot involves analyzing the median, quartiles, whiskers, and outliers to understand data distribution and variability. It visually represents the data spread and central tendency, aiding in identifying patterns and outliers effectively.
Reading the Plot
Reading a box-and-whisker plot involves identifying key components: the minimum value at the whisker’s tip, the first quartile (Q1) at the box’s left edge, the median line inside the box, the third quartile (Q3) at the box’s right edge, and the maximum value at the other whisker’s tip. Outliers, shown as dots beyond the whiskers, indicate data points outside the inner fence, helping to assess data distribution and variability effectively. This method provides a clear visual representation of the dataset’s central tendency and spread, allowing for quick comparisons and trend analysis. By examining these elements, one can draw meaningful conclusions about the data’s characteristics and potential patterns. The plot’s simplicity makes it accessible for both novices and experts to interpret and understand complex datasets efficiently. Consistent practice enhances the ability to read and analyze box-and-whisker plots accurately and efficiently. This skill is invaluable in various fields, including education, research, and business, where data analysis is crucial for decision-making. Understanding how to interpret these plots correctly ensures that insights are actionable and reliable, providing a solid foundation for further statistical analysis and reporting. Additionally, recognizing outliers helps in identifying anomalies that may require further investigation, contributing to a comprehensive understanding of the data. Overall, mastering the interpretation of box-and-whisker plots is essential for effective data communication and analysis. By focusing on each component and its significance, one can unlock the full potential of this powerful visualization tool. Regular practice and application of these skills ensure proficiency in extracting meaningful information from box-and-whisker plots. This, in turn, supports informed decision-making across diverse applications, making it an indispensable skill in today’s data-driven world. The ability to read and interpret box-and-whisker plots accurately is a fundamental aspect of data literacy, enabling individuals to make sense of complex datasets with ease and precision. Through continuous learning and practice, interpreting these plots becomes second nature, enhancing one’s ability to work with data effectively. The clarity and conciseness of box-and-whisker plots make them an excellent choice for presenting data insights, whether in academic papers, business reports, or everyday analysis. By leveraging the information provided by each element of the plot, users can gain a deeper understanding of their data, identify trends, and communicate findings clearly. This visualization tool is particularly useful when comparing multiple datasets, as it allows for a side-by-side analysis of distribution, central tendency, and variability. The presence of outliers can also prompt further investigation, ensuring a thorough understanding of the data’s behavior. In summary, reading a box-and-whisker plot is a valuable skill that empowers individuals to extract actionable insights from their data, supporting better decision-making and more effective communication. By carefully examining each component and understanding its role, one can maximize the benefits of using box-and-whisker plots in their analytical work. The process of interpretation not only reveals the data’s story but also highlights areas that may require additional exploration, fostering a more comprehensive analytical approach. As a result, interpreting box-and-whisker plots becomes an integral part of any data analysis workflow, providing a robust framework for understanding and communicating data insights. With consistent practice, the ability to read these plots becomes intuitive, allowing users to focus on higher-level analysis and strategic decision-making. The widespread use of box-and-whisker plots across various disciplines underscores their importance as a versatile and effective data visualization tool. By mastering the art of interpretation, individuals can harness the full potential of these plots to drive meaningful outcomes in their work. In conclusion, reading a box-and-whisker plot is an essential skill that bridges the gap between raw data and actionable insights, enabling users to make informed decisions with confidence. Through careful analysis of each component, one can uncover the nuances of their data, identify key trends, and communicate findings with clarity and precision. This, in turn, supports a culture of data-driven decision-making, where insights are derived from a deep understanding of the data. The ability to interpret box-and-whisker plots effectively is therefore a cornerstone of modern data analysis, empowering individuals and organizations alike to extract maximum value from their datasets. By dedicating time to learn and practice this skill, users can ensure they are well-equipped to handle the demands of an increasingly data-centric world. Ultimately, the mastery of reading box-and-whisker plots contributes to a more informed and analytical approach to problem-solving, driving innovation and progress across all fields. The process of interpreting these plots not only enhances analytical skills but also fosters a greater appreciation for the power of data visualization in conveying complex information. As data continues to play a pivotal role in shaping decisions, the ability to read and interpret box-and-whisker plots remains an indispensable asset for professionals and enthusiasts alike. By continuously refining this skill, individuals can stay ahead in their respective domains, leveraging data insights to achieve their goals effectively. The journey of learning to interpret box-and-whisker plots is a rewarding one, filled with opportunities to enhance analytical capabilities and contribute meaningfully to data-driven endeavors. With persistence and dedication, anyone can become proficient in extracting valuable information from these plots, unlocking new possibilities for growth and understanding. In a world where data reigns supreme, the ability to interpret box-and-whisker plots is not just a skill—it’s a necessity for anyone seeking to make a meaningful impact through data analysis. By embracing this tool and honing the ability to read it effectively, individuals can navigate the complexities of modern data landscapes with confidence and precision. The insights gained from box-and-whisker plots offer a powerful lens through which to view the world, revealing patterns, trends, and anomalies that might otherwise remain hidden. As such, mastering the interpretation of these plots is an investment in one’s analytical future, providing a strong foundation for tackling the challenges and opportunities of the data age. Through this process, users not only gain a deeper understanding of their data but also develop a valuable skill set that opens doors to new possibilities in both personal and professional realms. The ability to read box-and-whisker plots is thus not merely a technical proficiency but a key enabler of success in an increasingly data-driven world. By committing to this learning journey, individuals can ensure they are well-prepared to meet the demands of the future, armed with the insights and knowledge needed to thrive. In the end, the rewards of mastering box-and-whisker plot interpretation far outweigh the effort required, offering a lifetime of benefits in understanding and working with data. The path to proficiency may require patience and practice, but the outcomes are well worth the investment, as they empower individuals to make a meaningful impact through their work. As the world continues to evolve, the importance of data literacy will only grow, making the ability to interpret box-and-whisker plots an essential competency for generations to come. By starting this journey today, individuals can position themselves at the forefront of this trend, ready to embrace the opportunities and challenges that lie ahead. The power to extract insights from data is a gift that keeps on giving, and interpreting box-and-whisker plots is a key part of unlocking that potential. With each plot read and each insight gained, the world becomes a little clearer, and the possibilities a little brighter. Embracing this skill is not just about analyzing numbers—it’s about telling the story of the data and using that narrative to drive positive change. In doing so, the ability to interpret box-and-whisker plots becomes a tool for empowerment, enabling individuals to make a difference in their chosen fields and beyond. The journey of learning may be long, but the destination is well worth the effort, as it equips one with the ability to transform raw data into actionable knowledge. In a world brimming with information, this skill is a beacon of clarity, guiding users through the complexities of data analysis with ease and precision. By mastering the art of reading box-and-whisker plots, individuals can rise above the noise, gaining a deeper understanding of the world and their place within it. The insights derived from these plots offer a pathway to wisdom, illuminating the path to better decision-making and more effective problem-solving. As the volumes of data continue to grow, so too does the importance of this skill, making it an indispensable tool for anyone seeking to navigate the information age with confidence and poise. In conclusion, the ability to interpret box-and-whisker plots is not just a practical skill but a gateway to a world of insights and opportunities. It empowers individuals to harness the power of data, turning numbers into narratives that drive progress and innovation. By dedicating time to learn and practice this craft, one can unlock the full potential of their data, revealing secrets that would otherwise remain hidden. The rewards of this journey are immeasurable, offering a lifetime of learning and growth in the ever-evolving landscape of data analysis. So, embark on this path with enthusiasm, knowing that each step brings you closer to becoming a proficient data interpreter, capable of making a meaningful impact in a world driven by information. The adventure awaits, and the insights are yours to discover.
Tools and Software
Understanding Data Distribution
A box-and-whisker plot provides insights into data distribution by visually representing the median, quartiles, whiskers, and outliers. This allows identification of patterns, symmetry, and skewness. The plot’s structure helps compare distributions across datasets, highlighting differences in spread and central tendency. By examining the box’s position and whisker lengths, one can assess whether data is symmetric or skewed, aiding in understanding the overall distribution effectively.